在图中加入一个新节点及其相关边后,要更新最小生成树(MST),通常意味着需要重新计算包含新节点的最小生成树,因为新节点的加入可能改变了原有MST的结构。直接更新原有MST的复杂度可能很高,且不一定能保证结果正确,因此一般的方法是重新计算包含新节点和旧图的最小生成树。
下面是一个使用Go语言实现的简单框架,利用Kruskal算法(一种常用于寻找最小生成树的算法)来重新计算包含新节点和边的最小生成树。这里假设图以边列表的形式给出,每条边包括连接的顶点和权重。
首先,定义边和并查集的基本结构:
package mainimport("fmt""sort")typeEdgestruct{ u, v, w int}// 并查集typeUnionFindstruct{ parent []int rank []int count int}func newUnionFind(n int)*UnionFind{ parent :=make([]int, n) rank :=make([]int, n)for i :=0; i < n; i++{ parent[i]= i rank[i]=0}return&UnionFind{parent, rank, n}}func (uf *UnionFind) find(x int)int{if uf.parent[x]!= x { uf.parent[x]= uf.find(uf.parent[x])// 路径压缩}return uf.parent[x]}func (uf *UnionFind)union(x, y int)bool{ rootX, rootY := uf.find(x), uf.find(y)if rootX == rootY {returnfalse}if uf.rank[rootX]> uf.rank[rootY]{ uf.parent[rootY]= rootX}elseif uf.rank[rootX]< uf.rank[rootY]{ uf.parent[rootX]= rootY}else{ uf.parent[rootY]= rootX uf.rank[rootX]++} uf.count--returntrue}// Kruskal 算法func kruskal(edges []Edge, n int)[]Edge{ uf := newUnionFind(n) mst :=[]Edge{} sort.Slice(edges,func(i, j int)bool{return edges[i].w < edges[j].w})for _, e :=range edges {if uf.union(e.u, e.v){ mst =append(mst, e)iflen(mst)== n-1{break}}}return mst}func main(){// 假设这是旧图的最小生成树边 oldEdges :=[]Edge{{0,1,1},{1,2,2},{2,3,3}} n :=4// 旧图的节点数// 新加入的节点和边 newNode :=4 newEdges :=[]Edge{{0,4,5},{3,4,4}}// 合并新旧边 allEdges :=append(oldEdges, newEdges...)// 重新计算包含新节点的最小生成树 mst := kruskal(allEdges, n+1)// 节点数变为 n+1 fmt.Println("新的最小生成树边为:")for _, e :=range mst { fmt.Printf("(%d, %d, %d) ", e.u, e.v, e.w)}}上述代码首先定义了边和并查集的结构,然后实现了Kruskal算法来找到最小生成树。在main函数中,我们假设了一些旧图的边和新加入的节点及边,然后将它们合并后重新计算了包含新节点的最小生成树。注意,这里的n是旧图的节点数,新节点加入后,节点总数变为n+1。
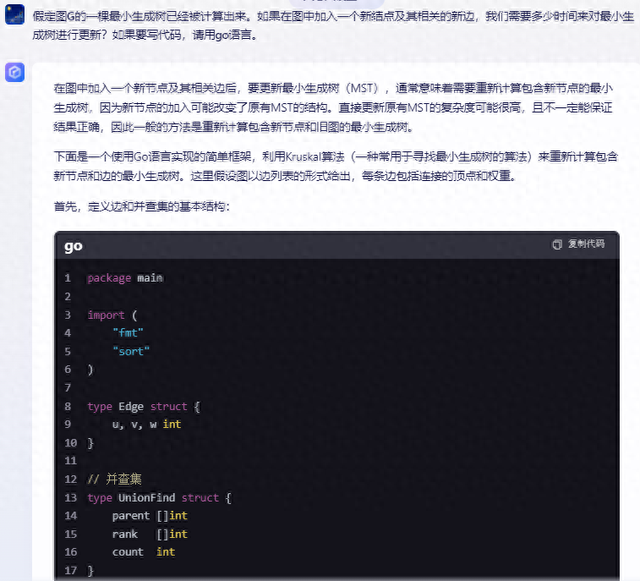
在这里插入图片描述
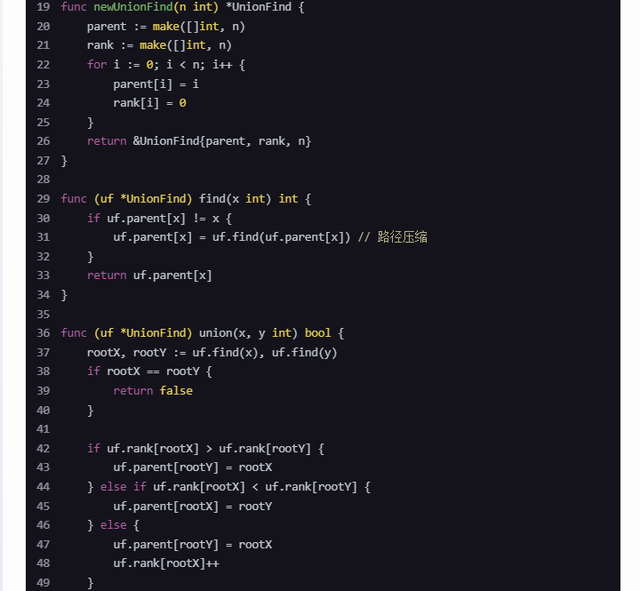
在这里插入图片描述
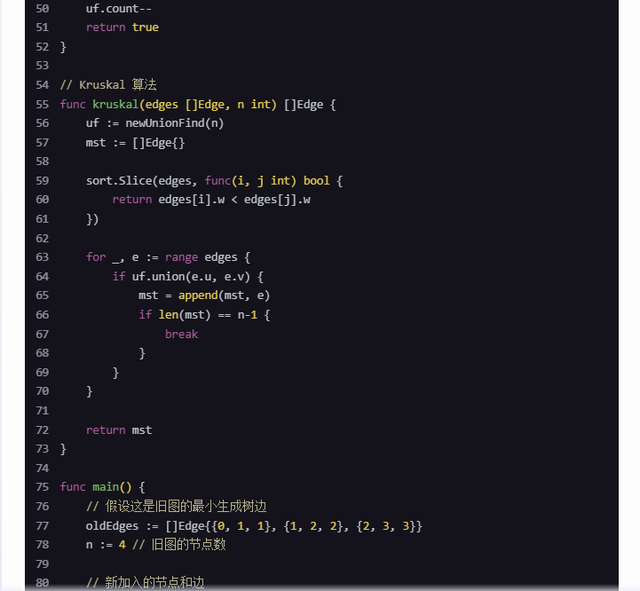
在这里插入图片描述
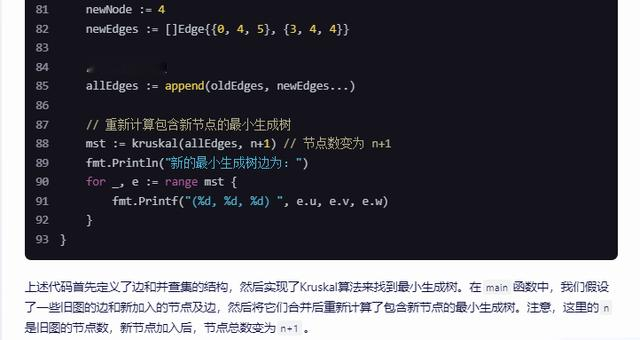
在这里插入图片描述
讯飞星火:在已经计算出最小生成树的情况下,如果图中加入一个新结点及其相关的新边,我们需要的时间取决于新加入的边是否会影响当前的最小生成树。如果新加入的边与最小生成树中的任何一条边形成了环,那么我们需要更新最小生成树。否则,新加入的边不会对最小生成树产生影响。
以下是使用Go语言实现的一个简单示例,用于检查新加入的边是否会导致环的形成:
package mainimport("fmt")typeEdgestruct{ src int dest int weight int}func find(parent []int, i int)int{if parent[i]==-1{return i}return find(parent, parent[i])}func union(parent []int, x int, y int)bool{ xset := find(parent, x) yset := find(parent, y)if xset != yset { parent[xset]= ysetreturntrue}returnfalse}func isCycle(edges []Edge, V int)bool{ parent :=make([]int, V)for i :=0; i < V; i++{ parent[i]=-1}for _, edge :=range edges {if!union(parent, edge.src, edge.dest){returntrue}}returnfalse}func main(){ V :=4// Number of vertices in graph including the new node E :=5// Number of edges in the original MST edges :=[]Edge{{0,1,10},{0,2,6},{0,3,5},{1,3,15},{2,3,4},}// Add a new edge between node 3 and a new node 4 newEdge :=Edge{3,4,8} edges =append(edges, newEdge)if isCycle(edges, V){ fmt.Println("The graph has a cycle after adding the new edge.")}else{ fmt.Println("The graph does not have a cycle after adding the new edge.")}}在这个示例中,我们首先定义了一个Edge结构体来表示图的边。然后,我们实现了find和union函数,这两个函数是并查集算法的一部分,用于检测图中是否存在环。最后,我们实现了isCycle函数,该函数接受一个边的列表和顶点的数量,然后使用并查集算法检测是否存在环。
在main函数中,我们创建了一个包含4个顶点和5条边的图。然后,我们添加了一条新的边,连接节点3和新节点4。最后,我们调用isCycle函数来检查添加新边后图是否形成环。
360智脑:在图G中添加一个新的节点和与之相关的边后,要更新最小生成树,我们可以使用Prim算法或Kruskal算法。这两种算法的时间复杂度在最坏情况下都是O(E log E),其中E是边的数量。但是,如果我们已经有了一个最小生成树,并且只是添加了一个新的节点和一些边,那么我们可以利用现有的最小生成树信息来加速更新过程。
以下是一个简化的Go语言代码示例,展示了如何使用Prim算法来更新最小生成树(假设我们已经有了一棵最小生成树):
import ("container/heap""math")typeEdgestruct{from, to int weight float64}typePriorityQueue[]*Edgefunc (pq PriorityQueue)Len()int{returnlen(pq)}func (pq PriorityQueue)Less(i, j int)bool{return pq[i].weight < pq[j].weight}func (pq PriorityQueue)Swap(i, j int){ pq[i], pq[j]= pq[j], pq[i]}func (pq *PriorityQueue)Push(x interface{}){*pq =append(*pq, x.(*Edge))}func (pq *PriorityQueue)Pop()interface{}{ old :=*pq n :=len(old) item := old[n-1] old[n-1]=nil// avoid memory leak*pq = old[0: n-1]return item}func updateMST(graph [][]Edge, mst []Edge, newNode int, newEdges []Edge)[]Edge{// Add new edges to the graphfor _, edge :=range newEdges { graph[edge.from]=append(graph[edge.from], edge) graph[edge.to]=append(graph[edge.to], edge)}// Initialize priority queue with edges from the new node pq :=make(PriorityQueue,0)for _, edge :=range newEdges {if edge.from== newNode { pq =append(pq,&edge)}elseif edge.to == newNode { pq =append(pq,&edge)}} heap.Init(&pq)// Add new node to the MST mst =append(mst,Edge{})// placeholder for the new node's edge// Update MST using Prim's algorithm visited :=make([]bool,len(graph))forlen(pq)>0{ edge := heap.Pop(&pq).(*Edge)if visited[edge.to]&& visited[edge.from]{continue}if!visited[edge.to]{ edge.to, edge.from= edge.from, edge.to} mst[newNode]=*edge visited[edge.to]=truefor _, neighborEdge :=range graph[edge.to]{if!visited[neighborEdge.to]{ heap.Push(&pq,&neighborEdge)}}}return mst}这段代码首先将新节点和新边添加到图中,然后初始化一个优先队列,其中包含所有从新节点出发的边。接着,它使用Prim算法来更新最小生成树。在这个过程中,我们假设mst切片已经包含了原始的最小生成树,并且我们有足够的空间来添加新的边。
请注意,这个代码示例是一个简化的版本,实际应用中可能需要更多的错误检查和优化。此外,如果新节点连接了很多其他节点,那么更新过程可能会比较耗时,因为它需要重新遍历所有的边。在这种情况下,可能需要更高效的算法或者数据结构来处理更新。
智谱清言:在图中加入一个新节点及其相关的新边后,更新最小生成树的时间复杂度取决于新边的数量以及最小生成树的当前结构。以下是更新最小生成树的步骤:
1. 将新节点加入最小生成树。2. 对于每条与新节点相连的新边,检查是否形成了一个环。如果形成环,则忽略这条边;如果没有形成环,则检查这条边是否可以替换当前最小生成树中的一条边以获得更小的总权重。这可以通过以下方式实现:• 使用Kruskal或Prim算法中的并查集数据结构来检查是否形成环。• 使用边的新权重与当前最小生成树中的最大边权重进行比较,以判断是否需要进行替换。 对于每条新边,这个检查过程的时间复杂度是O(logV),其中V是节点数。如果新节点有k条边,那么总的时间复杂度将是O(klogV)。 以下是使用Go语言实现更新最小生成树的一个简单示例:package mainimport("fmt")// Edge represents an edge in the graphtypeEdgestruct{FromintTointWeightint}// Graph represents a graph with vertices and edgestypeGraphstruct{VerticesintEdges[]Edge}// UnionFind is used to detect cycles in the graphtypeUnionFindstruct{Parent[]intRank[]int}// NewUnionFind initializes a new UnionFindfunc NewUnionFind(vertices int)*UnionFind{uf :=&UnionFind{Parent:make([]int, vertices),Rank:make([]int, vertices),}for i :=0; i < vertices; i++{uf.Parent[i]= iuf.Rank[i]=0}return uf}// Find finds the representative of the set that contains 'v'func (uf *UnionFind)Find(v int)int{if uf.Parent[v]!= v {uf.Parent[v]= uf.Find(uf.Parent[v])// path compression}return uf.Parent[v]}// Union merges the sets that contain 'u' and 'v'func (uf *UnionFind)Union(u, v int){rootU := uf.Find(u)rootV := uf.Find(v)if rootU != rootV {// Union by rankif uf.Rank[rootU]> uf.Rank[rootV]{uf.Parent[rootV]= rootU}elseif uf.Rank[rootU]< uf.Rank[rootV]{uf.Parent[rootU]= rootV}else{uf.Parent[rootV]= rootUuf.Rank[rootU]++}}}// AddVertex adds a new vertex to the graphfunc (g *Graph)AddVertex(){g.Vertices++}// AddEdge adds a new edge to the graphfunc (g *Graph)AddEdge(from, to, weight int){g.Edges=append(g.Edges,Edge{From:from,To: to,Weight: weight})}// UpdateMST updates the minimum spanning tree with a new vertex and its edgesfunc (g *Graph)UpdateMST(newVertex int, newEdges []Edge)[]Edge{uf :=NewUnionFind(g.Vertices)mst :=make([]Edge,0)// Add existing MST edges to the new MSTfor _, edge :=range g.Edges{if uf.Find(edge.From)!= uf.Find(edge.To){uf.Union(edge.From, edge.To)mst =append(mst, edge)}}// Add new edges to the MST if they do not form a cyclefor _, edge :=range newEdges {if uf.Find(edge.From)!= uf.Find(edge.To){uf.Union(edge.From, edge.To)mst =append(mst, edge)}}return mst}func main(){// Initialize a graph with some edgesgraph :=Graph{Vertices:4}graph.AddEdge(0,1,10)graph.AddEdge(1,2,6)graph.AddEdge(2,3,5)// Add a new vertex with edgesgraph.AddVertex()newEdges :=[]Edge{{From:3,To:4,Weight:2},{From:2,To:4,Weight:3}}// Update the MSTmst := graph.UpdateMST(4, newEdges)// Print the updated MSTfor _, edge :=range mst {fmt.Printf("Edge from %d to %d with weight %d\n", edge.From, edge.To, edge.Weight天工:更新最小生成树(MST)以适应新加入的节点及其相关边的时间复杂度主要依赖于使用的MST更新算法。一种直观的方法是将新节点与图中的所有节点相连(即加入所有新边),然后重新运行MST算法(如Prim或Kruskal算法)。但是,这种方法的时间复杂度较高,因为需要重新计算整个图的MST。
更有效的方法是使用增量算法。在图中加入一个新节点后,我们只需要检查与新节点相连的所有边,看这些边是否能构成更优的MST。具体来说,对于与新节点相连的每条边,我们检查这条边是否可以替换掉MST中的一条边,从而减少总权重。这通常涉及到维护一个优先队列(最小堆)来快速选择权重最小的边。
在Go语言中,实现这种增量更新的算法可能涉及以下步骤:
1. 将新节点加入图中。2. 对于新节点的每一条边,检查是否可以优化MST。3. 如果新边的权重小于MST中连接相同节点的边的权重,更新MST。4. 重复步骤3直到没有更优的替换。下面是一个简化的Go代码示例,展示如何使用优先队列更新MST:
package mainimport("container/heap""fmt")typeEdgestruct{from, to, weight int}typePriorityQueue[]*Edgefunc (pq PriorityQueue)Len()int{returnlen(pq)}func (pq PriorityQueue)Less(i, j int)bool{// We want Pop to give us the lowest, not highest, priority so we use less than here.return pq[i].weight < pq[j].weight}func (pq PriorityQueue)Swap(i, j int){ pq[i], pq[j]= pq[j], pq[i]}func (pq *PriorityQueue)Push(x interface{}){// Push and Pop use pointer receivers because they modify the slice's length,// not just its contents.*pq =append(*pq, x.(*Edge))}func (pq *PriorityQueue)Pop()interface{}{ old :=*pq n :=len(old) x := old[n-1]*pq = old[0: n-1]return x}func updateMST(mstEdges []*Edge, newEdges []*Edge)[]*Edge{ pq :=make(PriorityQueue,len(newEdges))for i, e :=range newEdges { pq[i]= e} heap.Init(&pq)for pq.Len()>0{ e := heap.Pop(&pq).(*Edge)// Check if this edge can improve the MSTfor _, mst :=range mstEdges {if(mst.from== e.from&& mst.to == e.to)||(mst.from== e.to && mst.to == e.from){if mst.weight > e.weight {// Replace the edge in MST mst.weight = e.weight}break}}}return mstEdges}func main(){// Example usage mstEdges :=[]*Edge{{0,1,1},{1,2,2},{2,3,3}} newEdges :=[]*Edge{{3,4,1},{4,0,2}} mstEdgesUpdated := updateMST(mstEdges, newEdges) fmt.Println("Updated MST Edges:", mstEdgesUpdated)}注意,这个示例代码是简化的,实际应用中可能需要更复杂的图表示和更详细的MST更新逻辑,包括处理图的连通性和循环。此外,对于大规模图,可能还需要考虑更高效的算法和数据结构。
代码中使用了Go标准库中的container/heap包来实现优先队列,这可以有效地处理边的权重,确保每次都能选择权重最小的边进行检查。
chatgpt:当在一个已有的最小生成树上添加一个新节点和相关的新边时,我们可以使用以下两种方法来更新最小生成树:
1. Prim's算法: 使用Prim's算法,可以通过在现有的最小生成树中找到连接新节点的最小权重边来更新最小生成树。这种方法的时间复杂度为O(V^2),其中V是图中节点的数量。2. Kruskal's算法: Kruskal's算法则是通过将新边加入现有的最小生成树并通过最小堆来找到合适的位置,以维持最小生成树的性质。这个方法的时间复杂度为O(ElogV),其中E是边的数量,V是节点的数量。下面是一个用Go语言实现Prim's算法更新最小生成树的简单示例代码:
package mainimport"fmt"typeGraphstruct{ vertices int adjMatrix [][]int}func (g *Graph)PrimMST(u int, key []int, parent []int){ key[u]=0 mstSet :=make([]bool, g.vertices)for count :=0; count < g.vertices-1; count++{ minKey :=int(^uint(0)>>1) minVertex :=-1for v :=0; v < g.vertices; v++{if mstSet[v]==false&& key[v]< minKey { minKey = key[v] minVertex = v}} mstSet[minVertex]=truefor v :=0; v < g.vertices; v++{if g.adjMatrix[minVertex][v]>0&& mstSet[v]==false&& g.adjMatrix[minVertex][v]< key[v]{ parent[v]= minVertex key[v]= g.adjMatrix[minVertex][v]}}}for i :=1; i < g.vertices; i++{ fmt.Println(parent[i],"-", i,"\t", g.adjMatrix[i][parent[i]])}}func main(){ g :=Graph{ vertices:5, adjMatrix:[][]int{{0,2,0,6,0},{2,0,3,8,5},{0,3,0,0,7},{6,8,0,0,9},{0,5,7,9,0},},} key :=make([]int, g.vertices) parent :=make([]int, g.vertices)for i :=0; i < g.vertices; i++{ key[i]=int(^uint(0)>>1)} g.PrimMST(0, key, parent)}这段代码演示了如何使用Prim's算法来计算最小生成树,并进行更新。在这个例子中,我们假设图是一个加权无向图,并且通过邻接矩阵来存储图的结构。
kimi:在图论中,最小生成树(Minimum Spanning Tree, MST)是给定一个带权重的无向图,找到一棵包含图中所有顶点的树,且树的边权重之和最小。当图G的最小生成树已经计算出来,并且要加入一个新的结点及其相关的新边时,更新最小生成树的时间复杂度取决于所使用的算法和数据结构。
1. 使用Kruskal算法:如果使用Kruskal算法,每次添加新边时,你需要将新边加入到边的集合中,并重新执行算法。时间复杂度为O(E log E),其中E是边的数量。2. 使用Prim算法:如果使用Prim算法,添加新边后,你可以将新结点加入到优先队列中,并继续执行算法。时间复杂度为O(E log V),其中V是顶点的数量。3. 使用Boruvka算法:Boruvka算法是另一种构建最小生成树的算法,它特别适合于稀疏图。每次添加新边后,可以将其加入到边的集合中,并重新执行算法。时间复杂度为O(E log V)。4. 使用Union-Find数据结构:如果你使用Union-Find数据结构来辅助构建最小生成树,添加新边后,你可以将其加入到边的集合中,并使用Union-Find来检查是否形成环。这种方法的时间复杂度可以优化到接近线性。对于代码实现,以下是一个使用Go语言的简单示例,使用Union-Find数据结构来更新最小生成树:
package mainimport("fmt""sort")typeEdgestruct{Weightint V1 int V2 int}typeUnionFindstruct{ parent []int rank []int}func NewUnionFind(n int)*UnionFind{ parent :=make([]int, n) rank :=make([]int, n)for i :=range parent { parent[i]= i}for i :=range rank { rank[i]=0}return&UnionFind{parent, rank}}func (uf *UnionFind)Find(v int)int{if uf.parent[v]!= v { uf.parent[v]= uf.Find(uf.parent[v])}return uf.parent[v]}func (uf *UnionFind)Union(v1, v2 int){ root1 := uf.Find(v1) root2 := uf.Find(v2)if root1 != root2 {if uf.rank[root1]> uf.rank[root2]{ uf.parent[root2]= root1}elseif uf.rank[root1]< uf.rank[root2]{ uf.parent[root1]= root2}else{ uf.parent[root2]= root1 uf.rank[root1]++}}}func (uf *UnionFind)Connected(v1, v2 int)bool{return uf.Find(v1)== uf.Find(v2)}func addEdgeAndUpdateMST(edges []Edge, newEdge Edge, uf *UnionFind)[]Edge{ edges =append(edges, newEdge) sort.Slice(edges,func(i, j int)bool{return edges[i].Weight< edges[j].Weight}) mst :=[]Edge{}for _, edge :=range edges {if!uf.Connected(edge.V1, edge.V2){ uf.Union(edge.V1, edge.V2) mst =append(mst, edge)}}return mst}func main(){// 假设edges是已经计算出的最小生成树的边集合 edges :=[]Edge{{Weight:1, V1:0, V2:1},{Weight:2, V1:1, V2:2},{Weight:3, V1:2, V3:3},// ... 其他边}// 创建并初始化Union-Find数据结构 uf :=NewUnionFind(4)// 假设有4个顶点// 添加新边 newEdge :=Edge{Weight:4, V1:3, V2:0}// 更新最小生成树 mst := addEdgeAndUpdateMST(edges, newEdge, uf)// 打印更新后的最小生成树for _, edge :=range mst { fmt.Printf("Edge (%d, %d) with weight %d\n", edge.V1, edge.V2, edge.Weight)}}请注意,这个示例代码是为了说明如何使用Union-Find数据结构来更新最小生成树,并不是一个完整的最小生成树算法实现。在实际应用中,你可能需要根据具体情况调整代码。
混元:在图G中加入一个新节点及其相关的新边后,更新最小生成树的时间复杂度取决于所使用的算法。以下是几种常见的方法及其时间复杂度:
1. Prim算法:如果使用优先队列(如二叉堆)来实现Prim算法,添加一个新节点并更新最小生成树的时间复杂度为O(log V),其中V是图中顶点的数量。2. Kruskal算法:如果使用并查集(Union-Find)数据结构来实现Kruskal算法,添加一个新节点并更新最小生成树的时间复杂度为O(E α(V)),其中E是新加入边的数量,α(V)是Ackermann函数的反函数,通常可以视为一个非常小的常数。3. Borůvka算法:这个算法在实际应用中不常用,因为它的时间复杂度较高。下面是使用Go语言实现的一个简单的例子,假设我们使用Prim算法,并且已经有了一个最小生成树的实现。我们将添加一个新的节点和一条新边,并更新最小生成树。
package mainimport("container/heap""fmt")// 定义边的结构体typeEdgestruct{ to int// 边的终点 weight float64// 边的权重}// 定义优先队列中的元素typeItemstruct{ value int// 顶点的编号 priority float64// 与该顶点相连的最小边的权重 index int// 元素在堆中的位置}// 实现一个最小堆typePriorityQueue[]*Itemfunc (pq PriorityQueue)Len()int{returnlen(pq)}func (pq PriorityQueue)Less(i, j int)bool{return pq[i].priority < pq[j].priority}func (pq PriorityQueue)Swap(i, j int){ pq[i], pq[j]= pq[j], pq[i] pq[i].index = i pq[j].index = j}func (pq *PriorityQueue)Push(x interface{}){ n :=len(*pq) item := x.(*Item) item.index = n*pq =append(*pq, item)}func (pq *PriorityQueue)Pop()interface{}{ old :=*pq n :=len(old) item := old[n-1] old[n-1]=nil// 避免内存泄漏 item.index =-1// for safety*pq = old[0: n-1]return item}// Prim算法的实现func prim(graph [][]Edge, start int)[]Edge{ n :=len(graph) mst :=make([]Edge,0, n-1) visited :=make([]bool, n) pq :=make(PriorityQueue,0) heap.Push(&pq,&Item{value: start, priority:0})for pq.Len()>0{ item := heap.Pop(&pq).(*Item) u := item.valueif visited[u]{continue} visited[u]=truefor _, edge :=range graph[u]{ v := edge.toif!visited[v]{ heap.Push(&pq,&Item{value: v, priority: edge.weight})}}iflen(mst)< n-1{ mst =append(mst,Edge{to: u, weight: item.priority})}}return mst}// 更新最小生成树的函数func updateMST(graph [][]Edge, mst []Edge, newNode int, newEdges []Edge)[]Edge{// 将新节点和边添加到图中 graph =append(graph,make([]Edge,len(graph)+1))for _, edge :=range newEdges { graph[newNode]=append(graph[newNode], edge)}// 使用Prim算法重新计算最小生成树return prim(graph, mst[0].to)// 假设mst[0].to是原始最小生成树的起始节点}func main(){// 示例图的邻接表表示 graph :=[][]Edge{{{to:1, weight:1},{to:2, weight:3}},{{to:0, weight:1},{to:2, weight:1}},{{to:0, weight:3},{to:1, weight:1}},}// 计算原始最小生成树 mst := prim(graph,0) fmt.Println("Original MST:", mst)// 添加新节点和新边 newNode :=3 newEdges :=[]Edge{{to:0, weight:2},{to:1, weight:4},} mst = updateMST(graph, mst, newNode, newEdges) fmt.Println("Updated MST:", mst)}请注意,这个例子是一个简化的实现,它假设新节点的编号是图中现有节点的最大编号加一。在实际应用中,你可能需要更复杂的逻辑来处理节点和边的添加,以及确保图的表示是正确的。此外,这个例子没有考虑更新已经存在的最小生成树边的情况,这在实际应用中可能需要额外的逻辑来处理。